Smell & Taste
Our brain perceives our molecular environment through two chemical senses, olfaction and taste, by triggering the activation of chemical sensors named olfactory and gustatory receptors. These receptors are differentially activated by a virtually infinite number of molecules suggesting a highly complex combinatorial code (Figure 1).
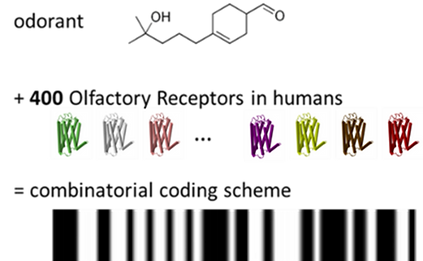
Figure 1: The brain perceives an odorant compound through differential activation of the 400 human olfactory receptors. The same combinatorial code exists for bitter taste involving 25 human bitter taste receptors.
Can a computer learn how to smell and taste? The objective of our research is to push the boundaries of machine learning models in the framework of chemical senses. Understanding how chemicals code for a certain type of percept is fundamental for basic research and for the food, flavor and fragrance industry. It would also be beneficial for the pharmacology industry and public health since scent detection in medical diagnosis by animals and electronic nose holds promise for the future.
In this context, the use of machine learning and deep learning models emerge as relevant methods to decode olfaction: The recent Dream Olfaction challenge has demonstrated that machine learning models, although not perfect, accurately predict odor qualities [1]. A team of researchers from Google Brain has successfully trained Graph Neural Networks (GNN) to predict odor descriptors [2,3]. We have recently shown that the application of machine learning in the context of virtual screening opens up the possibility of enlarging insect [4,5] and mammalian odorant [6] and taste chemical spaces [7]. Recently, we have also shown that deep learning models are able to predict the active OR-molecule pairs [8]. The originality of our approach lies in the combination of methods used: i) to encode the topology of an odorant molecule in the form of a molecular graph (GNN), i.e. by considering the atoms and the bonds respectively as nodes and edges of the network ; ii) the structural information of receptors extracted from their sequences using a strategy inspired by the field of natural language processing (NLP such as BERT or its variants). These recent results reinforce the hypothesis that we may be able to predict the olfactory quality of a given molecule by describing its chemical structure and its interactions with olfactory receptors.
[1] Predicting human olfactory perception from chemical features of odor molecules. A. Keller, R.C. Gerkin, Y. Guan, A. Dhurandhar, G. Turu, B. Szalai, J.D. Mainland, Y. Ihara, C.W. Yu, R. Wolfinger, C. Vens, l. Schietgat, K. De Grave, R. Norel, DREAM Olfaction Prediction Consortium, G. Stolovitzky, G.A. Cecchi, L.B. Vosshall, P. Meyer. Science 2017, 355, 820-826.
[2] Machine Learning for Scent: Learning Generalizable Perceptual Representations of Small Molecules. B. Sanchez-Lengeling, J.N. Wei, B.K. Lee, R.C. Gerkin, A. Aspuru-Guzik, A.B. Wiltschko. arXiv:1910.10685 [stat.ML], 2019.
[3] A Principal Odor Map Unifies Diverse Tasks in Human Olfactory Perception. B.K. Lee, E.J. Mayhew, B. Sanchez-Lengeling, J.N. Wei, W.W. Qian, K. Little, M. Andres, B.B. Nguyen, T. Moloy, J.K. Parker, R.C. Gerkin, J.D. Mainland, A.B. Wiltschko. bioRxiv 2022.09.01.504602, 2022.
[4] Machine learning decodes chemical features to identify novel agonists of a moth odorant receptor. G. Caballero-Vidal, C. Bouysset, H. Grunig, S. Fiorucci, N. Montagné, J. Golebiowski, E. Jacquin-Joly. Sci. Rep 2020, 10, 1655.
[5] Reverse chemical ecology in a moth: machine learning on odorant receptors identifies new behaviorally active agonists. G. Caballero-Vidal, C. Bouysset, J. Gévar, H. Mbouzid, C. Nara, J. Delaroche, J. Golebiowski, N. Montagné, S. Fiorucci, E. Jacquin-Joly. Cell. Mol. Life Sci., 2021, 78, 6593-6603
[6] Agonists of G Protein-Coupled Odorant Receptors are Predicted from Chemical Features. C. Bushdid, C.A. de March, S. Fiorucci, H. Matsunami, J. Golebiowski. J. Phys. Chem. Lett. 2018, 9, 2235-2240.
[7] Novel scaffold of natural compound eliciting sweet taste revealed by machine learning. C. Bouysset, C. Belloir, S. Antonczak, L. Briand, S. Fiorucci. Food Chem. 2020, 324, 126864.
[8] Matching receptor to odorant with protein language and graph neural networks, M. Hladiš, M. Lalis, S. Fiorucci, J. Topin. International Conference on Learning Representation (ICLR), 2023, online.